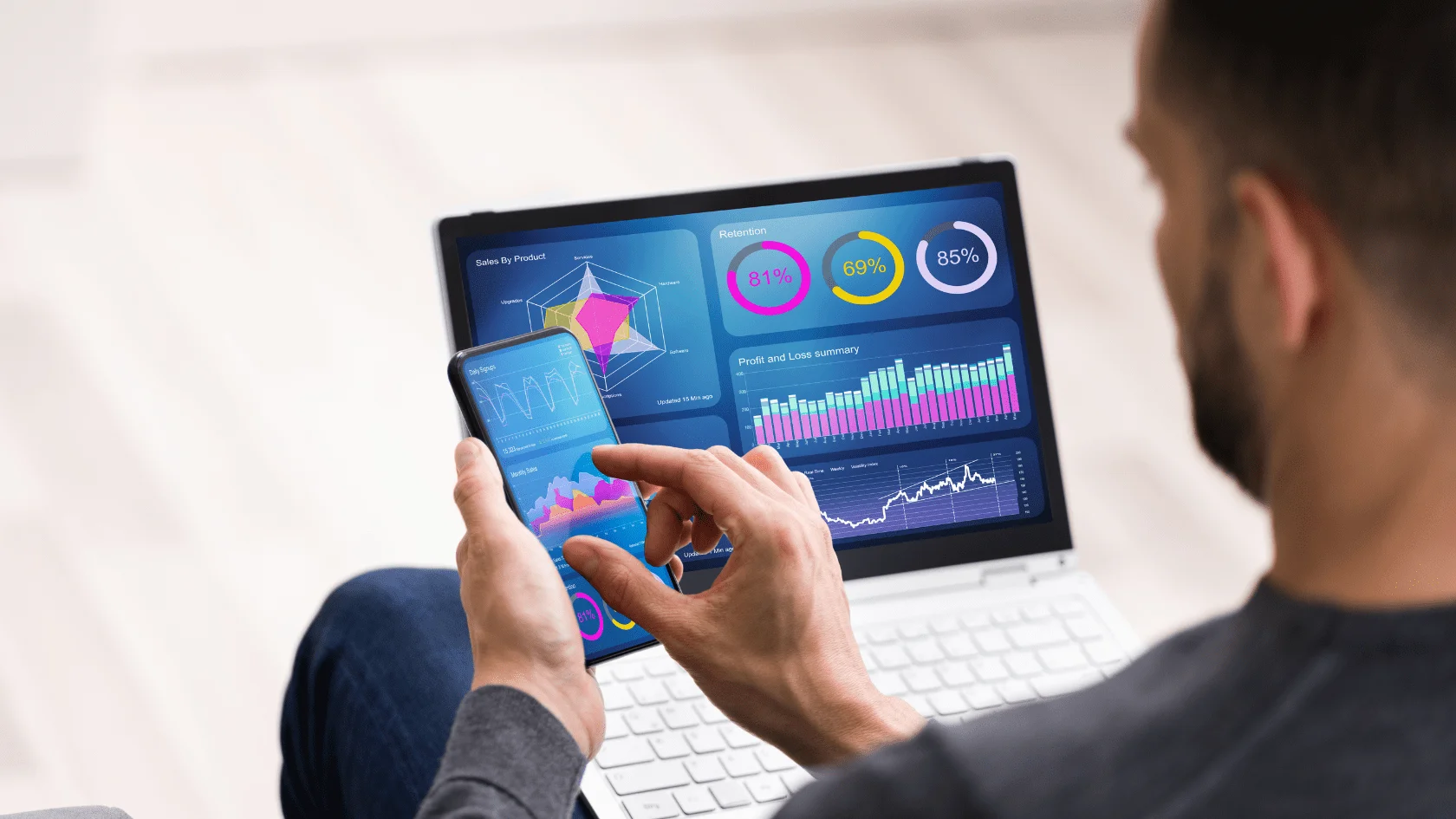
4 Roadblocks Hindering The Promise of Predictive Analytics
Large, complicated, and rapidly changing datasets from a variety of sources, including patient health records, real-time operational records, and claims information, are flooding healthcare systems. Predictive analytics not only aids in managing this deluge of data, but also in capitalizing on it, allowing for more informed, performance-enhancing decisions to be made.
Descriptive and diagnostic analytics help us understand “what happened” and “why did it happen,” respectively. Predictive analytics utilize statistical modeling, data mining, and machine learning to help us anticipate future events. Both also lay the groundwork for prescriptive analytics, through which we can prescribe precise actions based on past and present data.
Syntellis Performance Solutions recently surveyed healthcare finance executives, and 46% of those polled said they plan to use predictive analytics to help them and their patients make better decisions. Why isn’t this technology used in every hospital system when the value proposition is obvious and highly favored by executives? The short answer is that implementation is being slowed by a number of obstacles.
Data Illiterate Workforce.
According to Qlik’s survey, only 32% of C-suite directors are considered data literate. It’s possible that healthcare system leaders lack the knowledge and abilities necessary to implement predictive analytics. Users may need extensive data literacy training and adequate onboarding before they can make effective use of the technology.
Lag in Cloud Migration.
Cloud platforms can help enterprises improve patient care, security, and data-driven care. Organizations that are slow to migrate to the cloud may also miss out on predictive analytics’ full potential. Organizations who are still predominantly on-prem, mostly owing to HIPAA or other privacy issues, are at a disadvantage because many development initiatives are cloud-focused.
Interoperability Issues
Interoperability unites healthcare organization functions. Clinical, financial, and other data sets may reside in disparate systems and formats due to healthcare data interoperability issues. Predictive analytics may require interoperability improvements before implementation.
Overcoming Algorithmic Bias
For a long time, AI has been plagued by algorithm bias, and now people are worried it could make societal injustices even worse. Data used in predictive analytics must account for these biases or else the practice could cause more harm than good.
Predictive analytics has great potential, but overcoming these limitations is difficult. Healthcare firms should invest in more expert research and data-driven solutions to modernize and change care delivery.
Source: HITconsultant
🔥 Trending Stories
14 Tech Leaders Offer Their Best Pieces of Advice to New Entrepreneurs
Ultimate Guide For Hiring On-demand Developers For Your Startup
Top 25 Digital Transformation Influencers You Need to Follow